Share this
Become a stickler for the details in your research data
by Infotools on 20 Feb 2019
The devil is in the details! Our Group Client Director, Lena Cox, talks about the lessons she has learned about the importance of data protocol from working with big global brands like Coca-Cola.
There are times when you want someone to paint with broad, messy, rainbow strokes. This kind of unbridled creativity has a critical role to play in market research. Thinking outside the box, curiosity and passion are all essential qualities. But equally, we need a stickler with a check-list — someone with an eye for detail, who knows the rules and enforces them.
When it comes to data, we always want a strict protocol to ensure data quality. Because let's face it, it doesn't matter how great your analysis is—or how fancy the visualizations you share are—if the core underlying data isn't reliable and accurately reflect the market. At Infotools, we implement protocol and data quality for many of our clients and believe this step should be a "non-negotiable" for successful market research outputs. For clients who participate in things like global tracking programs across multiple markets, this approach is increasingly crucial for consistency.
Over the last three decades, I've learned many lessons about how changes to survey methodology can impact data quality and the ability to uncover market trends. These lessons ring especially true when working with clients who own multiple brands, operate across borders, and have many stakeholders along the research continuum. For example, Infotools works with The Coca-Cola Company, which owns more than 500 brands in more than 200 countries. Here are some tips based on our work with Coca-Cola and other clients:
1. Involve data expertise in the early stages of complex research design implementation
Clever survey design is even more difficult and complicated when you're aiming for maximum participant engagement by ensuring ease of use and minimal time spent. Survey design is a vital piece, but data processing is just as necessary. Failing to account for complex scripts in the final processes can mean that the collected data doesn't translate into the final data output correctly. The impact of this can be substantial.
To illustrate this, imagine building a pyramid representing brand equity (where the goal is to identify which brands have the strongest affinity with consumers – i.e., are the favorite/preferred in their category). For a brand with multiple sub-brands—let's say Grape Co. also owns Red Grape Co., Green Grape Co., and Vitamin Co.—it's easy to miss a step in your survey coding. Let me explain: If Question One is, "What is your favorite fruit brand?" and the survey participant answers, "Grape," your coding will list 'Grape Co.' as their favorite brand. However, what if the follow-up question is, "What type of Grape were you thinking of?" and they answer, "Vitamin Grape"? Have you coded the survey data to make sure 'Vitamin Grape Co.' is now listed as their favorite brand? It sounds like a small detail, but when you look at the big picture, failing to make this distinction could result in the conclusion that 'Grape Co.' is the favorite brand, when actually 'Vitamin Grape Co.' could be more accurate.
2. Minimize brand list revisions
Managing the demands of survey-data users can be a big job for many clients. If a company has multiple brands in the market, stakeholders can often disagree about the importance of one brand or another. Long brand lists impact the quality of the data collected and the experience for the survey participant, who may feel overwhelmed if asked about too many different brands at once.
For example, if a company like Coca-Cola is conducting global research, they have a high number of brands that they want to explore. From its flagship cola to Disani water, Powerade, Minute Maid, and so many more, it's easy to see how lists can get overwhelming. Add this to naming challenges across borders—think Diet Coke and Coca-Cola Light—and it becomes clear that parameters need to be set up ahead of time.
We can start to overcome these kinds of challenges by following these recommendations:
- Create and follow strict criteria of both when a brand can be removed from a study OR is considered important enough to be included in a study.
- Follow strict quotas on the maximum number of brands that can be included in any particular survey.
- Minimize the number of times the survey can be changed to increase the chance of identifying trend breaks. Where possible, calendarize brand list reviews to a couple of times a year to match up with periods when information is required for brand planning.
3. Be wary of the impact of logo design
Logo design and sizing changes can majorly impact survey data collection. Sometimes, even well-known brands plummet in claimed usage following what was considered a simple change to the logo.
For example, when one of Infotools' client's brands dropped drastically in the charts, we took a look at the logo within the survey. On review, the brand font size was so small, it was almost unreadable. It's no wonder the survey participants did not recognize it!
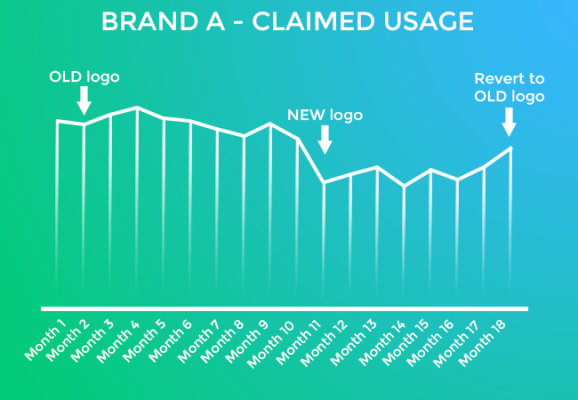
The impact of a logo change to a very well known brand, revert to old logo, and trend returns immediately back to previous levels. Sales remain stable in the market.
When using logos, it's critical to ensure no one image is causing bias to others within the survey. Make sure they're all set to the same size so that none dominate and that all are easy to read and recognize.
Another surprisingly common issue is discovering a mismatch between the logo used by the market research team compared to the logo, which is used in the market. You'd be surprised how often there is a disconnect between the marketing team and the market research team. So check what's actually out in the market and ensure the logo in your survey reflects what consumers are seeing.
4. Good sampling design and weighting is crucial
Some markets say, "We don't need to weight the data because we're applying strict quotas to our data collection."
But with a bit of digging, we often discover that there actually was some flexibility around quotas. Although small, these cause minor fluctuations in the consistency of the sample over time. Even if the weights only have a minor impact due to the quotas used, they should still be applied. Small fluctuations can impact the reliability of trend changes, multiplying over time to be much more significant, especially when overlaying population projection on the data. By weighting, we remove any risk the small fluctuations are impacting the insights.
5. Check, check, and recheck your script!
If your survey design is not implemented correctly in your script, then the impact can be very significant. The most common issue I see is the failure to implement brand rotation as specified in the design—which can be critical to even representation of the brands in the market.
The other key issue, which is becoming more important as increased complexity is included in survey design, is the correct inclusion of skips through the survey. If a skip isn't set up correctly early in a script, then the knock-on effect to later can be dire. So check, check and recheck the script against the questionnaire design to ensure all instructions have been included. And for global studies, you want a global script (i.e., all use the same tool), not just a globally shared document.
I know it sounds almost too basic, but always test the questionnaire from a survey participant's point of view. Put yourself in their shoes to see how they might react to what they see. Or better still, find a non-researcher to experience the survey and give feedback before it goes live.
As you can see, when it comes to data governance, you must be a stickler for the little things. This means being on the ground, knowing the ins and outs of survey data and market research. Sometimes it can mean challenging the status quo or putting your foot down to ensure the final results are robust. I believe it's critical for effective market research and makes all the difference to the final insights. So check in with your organization, who is the stickler with the checklist? The person you hate approaching when you're in a rush because you know they will force you to consider the little flaws in your plans? Go and talk to that person. Because now you know those small flaws can turn into costly mistakes down the line. (And if no one springs to mind, talk to the team at Infotools. We're handy with a checklist, and we know market research data inside out.)
Share this
- January 2025 (2)
- December 2024 (5)
- November 2024 (6)
- October 2024 (4)
- September 2024 (4)
- August 2024 (6)
- July 2024 (7)
- June 2024 (4)
- May 2024 (7)
- April 2024 (6)
- March 2024 (3)
- February 2024 (8)
- January 2024 (3)
- December 2023 (6)
- November 2023 (5)
- October 2023 (3)
- September 2023 (8)
- August 2023 (4)
- July 2023 (6)
- June 2023 (6)
- May 2023 (3)
- April 2023 (6)
- March 2023 (6)
- February 2023 (4)
- January 2023 (2)
- December 2022 (2)
- November 2022 (8)
- October 2022 (6)
- September 2022 (6)
- August 2022 (7)
- July 2022 (5)
- June 2022 (6)
- May 2022 (5)
- April 2022 (4)
- March 2022 (8)
- February 2022 (7)
- January 2022 (1)
- December 2021 (2)
- November 2021 (2)
- July 2021 (4)
- June 2021 (2)
- May 2021 (4)
- April 2021 (2)
- March 2021 (5)
- February 2021 (3)
- January 2021 (3)
- December 2020 (1)
- November 2020 (5)
- October 2020 (2)
- September 2020 (5)
- August 2020 (4)
- July 2020 (4)
- June 2020 (1)
- May 2020 (3)
- April 2020 (6)
- March 2020 (3)
- February 2020 (4)
- January 2020 (2)
- December 2019 (4)
- November 2019 (4)
- October 2019 (3)
- September 2019 (2)
- August 2019 (4)
- July 2019 (5)
- June 2019 (2)
- May 2019 (4)
- April 2019 (4)
- March 2019 (2)
- February 2019 (4)
- January 2019 (3)
- December 2018 (5)
- November 2018 (2)
- October 2018 (1)
- September 2018 (3)
- August 2018 (5)
- June 2018 (4)
- May 2018 (4)
- April 2018 (3)
- December 2017 (1)
- November 2017 (2)
- October 2017 (1)
- September 2017 (3)
- August 2017 (2)
- June 2017 (2)
- February 2017 (2)
- January 2017 (2)
- December 2016 (2)
- September 2016 (1)
No Comments Yet
Let us know what you think